Replay: Achieving Better Network Performance in the Age of HPC & AI
Traditional networks were never built to handle the demands of advanced computing workloads.
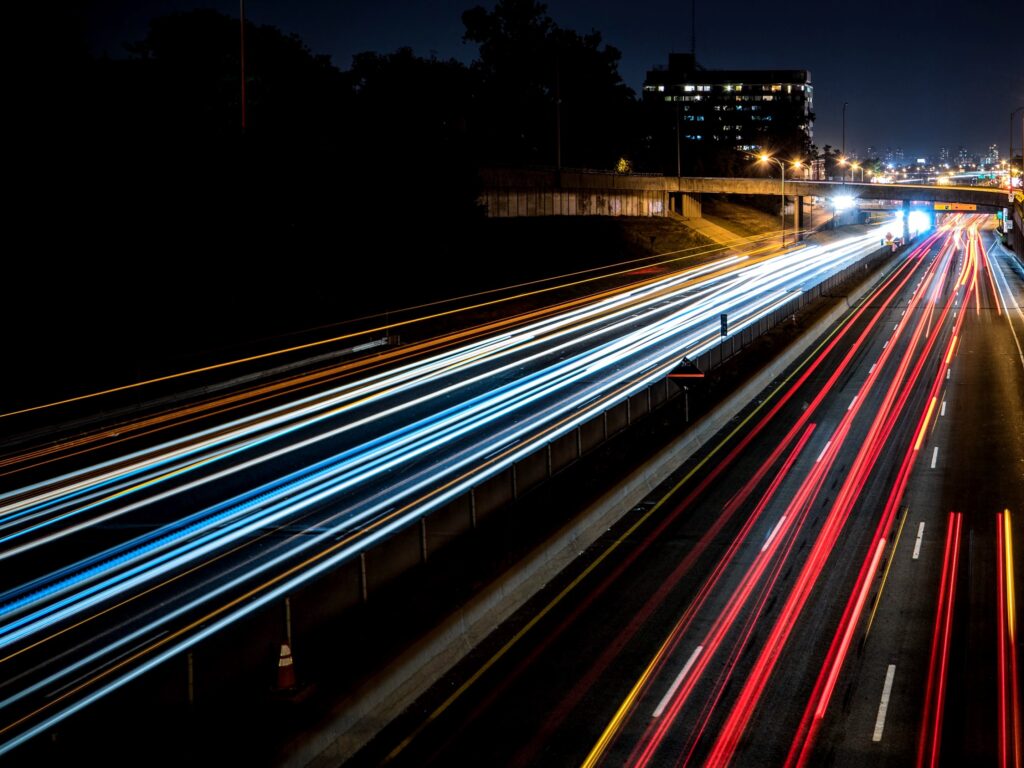
Watch the replay of this webinar (presented Jan. 27, 2022) for an overview of Rockport’s new approach to networking, built to meet the requirements of the converged era of HPC and AI:
- Better performance for more efficient data center utilization
- Less complexity with simple deployment and management
- Reduced power, cooling, and space for a greener data center
Video Transcript
We’re going to get started now, and thank you, everyone for taking a little bit of time. What we’re going to do is we’re going to talk a little bit about how you can achieve better performance in the current age of HPC and artificial intelligence. And by that, we’re going to basically make an introduction to a new company in the industry, called Rockport Networks, and the Rockport Switchless Network.
If you have any questions, feel free to e-mail us at info@rockportnetworks.com.
By way of introduction, my name is Loy Evans, I’m the Director of Field Engineering for Rockport, and I’ve been in the IT industry for a long time. I’ve been doing networking and infrastructure work for over 25 years. I’ve done routing, switching IT, IP telephony, security, coding, and have decades worth of working on infrastructure design, operations, and management. I’ve worked for and had certifications in a number of different companies.
But the reason why I joined Rockport was that this is a very interesting technology, and a very new way to look at networking as a whole. And so, it was a very interesting and appealing thing for someone who’s had a background in networking for a long time to see something that’s really innovative and new in the market.
By way of agenda, what we’re going to talk about is how Rockport is actually going about the process of rethinking the network. With that, we’re going to have a little bit of a discussion about the impact of congestion and how that can have an effect on workloads and workload performance. Then we’ll talk about Rockport networking and some of the components that make up our solution.
We’ll also talk a little bit about the benchmarks and the performance that we’re seeing in our testing. Then we’ll transition and have a very quick discussion about the economic and operational benefits that we see from running a Rockport network.
After that, we’ll do a quick summary, then a Q&A and we’ll see if we can get those questions answered.
All right, so I’m going to do a quote the IDC from 2021 in their AI practice:
“The convergence of AI, data analytics and HPC has led to a new category called performance intensive computing. Advances in compute and storage are up ending infrastructures creating demand for faster in interconnects and faster networks.”
And the way that I interpret this is that basically what they’re saying is that the types of applications and the way applications are being run has really kind of created this new category.
And then that new category, unfortunately, is still being connected with the exact same type of networks that we have used over the past few decades.
And really, nobody has actually gone in and tried to think, or rethink how the network is being created and utilized for these types of environments.
So that is exactly what Rockport’s doing. We are rethinking the network with these types of things in mind.
So if you think back in the history of IT, I like to understand where things have come from. And if you understand a little bit about the history of data center architectures, and in specific, what the most common architecture is nowadays, which is spine leaf, the spine leaf architecture actually came about in the fifties.
It derived from a public switched telephone network, and now, in the industry, a number of different companies have enhanced and increased the performance of individual component tree. But the architecture itself has really not changed at all since the fifties.
And so what we end up with, which is what I like to term the 49th port problem, which is if you’ve got a single switch with 48 ports in it, and you want to add that 49th server, when you’re running a spine leaf architecture, you cannot just add one more server.
You’ve actually got to add a pretty significant amount, more infrastructure in the top of rack and in the row or middle of row switches. And when you have to acquire that, that that extra infrastructure, that’s where you hit the first, what I call a stair-step function in the cost of acquisition of that equipment.
Once you get that equipment in, then you end up running into secondary stair-step functions where, you have a lot more equipment, a lot more cabling, you have more facilities requirements in the power and cooling. And so you end up with another stair-step function in the complexity. And then from that complexity, the administrative overhead that it requires to manage that.
It also just so happens that, a performance of a network within a single switch is probably some of the best that you can get. However, once you have to scale it to go to a second layer excuse me, a second tier of switching in order to go from switch to switch, then latency actually becomes a stair-step function that actually comes into the mix.
And so what you end up with is a compounded cost and complexity model. That basically means that we are inefficiently using the resources that we have in the compute and storage environment. We end up with excessive things that take up really high dollar footprint in the data center and in the actual cooled racks, which means that we have unproductive use of the expensive data center real estate.
And on top of that, we’ve got some pretty significant challenges in design operations management of networks like this. Now we also, when we try to address this we’re, from Rockport, we’re trying to rethink things that will fix all the problems that we had in, in the traditional architecture. But we also wanted to put very specific interests in the latency that comes from congestion. So when I talked about the latency that comes from building a multi-tier or a spine leaf architecture, essentially what we see is that what happens is that certain types of workloads can actually get caught up in over congested links and buckets.
What that then causes a degradation of the workload and that they perform, they have a lower performance for getting workloads done. What that means is that when you invest in one of these performance intensive clusters, you end up paying for something, but getting a reduced amount of workload capacity out of what your investment is.
Which then actually is a, a result is you end up with unnecessary cluster and inefficiencies where you end up having idle resources that are waiting on things that are being stuck in congestion and latency, that essentially what that means is that what you’re paying for, you’re not getting the full investment out of that.
And you end up with excessive waste in the money that you spent in CAPEX and OPEX. To give you a concrete example of where we see a direct impact of latency for application workload completion times that then mean you’re basically have wasted time is if you look at there’s a thing called tail latency, and essentially what that means is that if you look at latency over a scale of zero to 100% of all the measurements. This example has 25 nodes in a compute cluster. That’s running say a parallel workload in this case, maybe it’s an HPC workload. That’s running in MPI collective that requires that all the nodes respond back with their step of the function being completed before the application code can go to the next step of the function.
So in this case, that, the latency spike basically puts our average, if we went from zero to a hundred, maybe the average is about maybe 40. I think if I remember calculation on that, it’s about 41 microsecond latency for the average, the problem is that 24 of these 25 nodes respond within the first 35 to 40 milliseconds microsecond range.
The problem is that the application step cannot go to the next step until all 25 have responded. And in this particular case, maybe one of these nodes actually got stuck in a buffer on an uplink that actually is waiting for a whole bunch of other large data flows to pass. And so maybe that one actually ends up with a tail latency of 200 microseconds.
The application could have finished in about 40 microseconds, but it ends up taking five times longer on that one particular step. Let’s imagine that this is an application that has a hundred of those steps. You can see that if we had, if everything ran on the 50th percentile, we could get those hundred steps done in 400 microseconds.
The problem is that because of tail latency and we have to wait for everybody to finish. We end up with idle nodes that are stalled and waiting for the 25th node to finish. And the application step would take 2000 microseconds. Now, imagine if that actually was another application that you had to run, maybe 2000 of those steps are assumed to 2000 of these functions with a hundred steps in each function. That’s where you can really start seeing the direct impact of tailing and see on overall application performance.
So what we’re doing to address this as Rockport is introducing something that we’re calling the Rockport switchless network. Now truth be told it is actually not a switchless network, but what we’ve done is that the specifically physical switches that go in top of rack and in the row, we’re removing those from the picture.
So we’re basically taking the function that those ran we’re dis-aggregating that. Then we’re putting it on a card that we embed directly into the compute nodes that are part of the cluster. So what this gives us is a smart networking card that has the ability to directly connect to up to 12 directly connected neighbors with a fully distributed routing and control plane that allows for much more linear scaling.
It gives us a much higher path diversity for communications, and it also gives us a much lower blast radius. So if we happen to have a hardware failure, this is not like losing a switch where we lose an entire racks worth of data. We would only have an impact of one twelfth of the total bandwidth of all of the directly connected neighbors.
And every one of those neighbors can automatically and very quickly route around the problem. So what we talk about here at Rockport is it’s a three-step process to building a Rockport network. Step one, you install the card in the server, step two you connect the cable to the card, and step three, you install the network manager.
So essentially these I’m going to go through each of the individual parts. And essentially what we have here is a PCI based card that has basically two functions to it. One of them is the host interface, which is a standards-based Ethernet interface. The operating system sees nothing, there is no custom requirement for the end user to have to install any custom drivers, no kernel loadable modules. There is literally no impact to the system whatsoever. It doesn’t know that there’s anything other than Ethernet interface.
The FPGA, which is the second stage of this, is where really all of the magic happens. So the magic actually happens in that FPGA. And we’ll get into some of the function of how the fabric works in a little bit, but essentially what that does is it only takes power from the host via the PCIE bus.
Then what it does is it figures out the routing, the forwarding and it cuts things up into sub frame assemblies called flits, which we’ll get into a little bit in a little bit later then after it leaves the FPGA, it’s nothing but light signals.
So that’s when we get to the next box or the second stage in this in this network is we connect to what’s called a SHFL, which we have a single cable, which is a, if you’re familiar with this, it’s an MTP 24 or an MPO 24 cable, which is a single cable with 24 individual fibers in it. Those 24 fibers are actually connected into 12 receive transmit pairs. And if you remember, I talked about just a little while ago that every card connects directly to up to 12 directly connected neighbors, and that’s how we do it. That goes into this shuffle. And if you notice, if you look, this is really not much more than just an optical a fan-out, right? It’s an optical interconnect that does not have any logic does not require any power and does not require any cooling.
And so it’s a very simplistic environment, although, what we have wired in this box is a pre-wired six dimensional torus. And if you’re not familiar with six-dimension torus, I’ll talk a little bit about what toruses look like in a little bit, but it’s basically a very advanced networking topology, but we’ve taken the complexity in and of the typology and the wiring away from the end user. You literally plug in one cable and you’re done and you automatically have access to a very advanced typology in the box.
The next step is the autonomous network manager. Now, one thing to note is the ANM is not like a traditional InfiniBand network where you have to have a subnet manager in order for the network to work. This is really an, a user interface that’s focused on day two operations. So we’ll spend a little bit of time digging into a little bit more of that. So the autonomous network manager is a, an intuitive management and visualization tool for a very what could be a very complex network. And so what our goal with the design of this interface was to make it much more simple, to understand and have situational awareness of what the network is doing, how the network is behaving and where we see problems.
So it is a time-based database or a what’s called a time series database, where we actually keep full metrics for up to seven days with extremely low granularity. So we can get down into the, the, second level intervals of when we observe something it’s got a restful API. We have the ability to export SNMP traps from this, so that if there is an existing network operation center that happens to be a part of the environment. We can send alerts to that where it alerts people to know, okay, we need to go look into ANM to see what’s going on. And now, again, as I mentioned before, there’s zero requirement of this to be up for network operation.
As a matter of fact, you can see up there in the top, right? A partner gave us some feedback from one of their customers that okay, they shut down the network management, but the network kept on running, which is an extremely big deal. And it’s a very different departure from what we see in other types of network like this, wherever there’s a subnet manager requirement.
So when you first log in, essentially what you get is a overall network health screen. That if you notice you’ve got a bunch of small dots, but then you’ve got a couple of dots that are bigger in there. And essentially what it is from a purely a design perspective, we want it to bring attention to the things that need attention immediately.
So whenever there’s a problem on a node the nodes that have the problem actually are enlarged in size centered in the graph and actually are highlighted with where issues are recurring. So what this allows us to do is we can log in and almost instantaneously have an idea of what’s going on overall in the network.
And what you’re looking at here is a 228 node network where we’ve got two nodes that actually have problem. Now once we actually say, okay, Hey, we’ve got a couple of notes that I have a problem from this interface. I don’t have to go to another spot. I can literally just go to that thing that has a problem, click on it and drill down into a node level view.
That node level view gives me a lot of really rich information about detailed data flow information. I can see things like alarms events. I can see optical, subsystem signal integrity. I can see where I have a CRC error and I can differentiate data flow based on what’s actually bound for that host.
And what’s actually transiting through this node. So it allows me to differentiate things that are actually, locally significant traffic and what’s actually passing through and I get the ability to take this and drill down one step farther where I can see minute detail about packet level information, types of traffic QoS size and types of packets.
And again, I have the ability to differentiate this between what’s ingress, what’s egress, what’s transit, and what actually is bound for the host that this card is inside of. Every single one of these things can be lined up to a seven day history along an interactive timeline. I can look at what’s currently live, or if I have a problem, I can basically pause time and I can go back and look at the particular point in which I had a problem and I can see what happened before, during, and after an issue from all these different levels of telemetry.
So now, to talk a little bit about just how the fabric works as a whole. So I mentioned that we have a two-sided card, Ethernet interface that, the host uses to send data to, then that interface, since Ethernet frames to our FPGA, the FPGA actually is where the magic happens, that’s where we have our intellectual property and we are running, what’s called the re Rockport network operating system or rNOS. So rNOS is actually what runs on the FPGA and it is responsible for doing all of the work, right? So the network card itself runs the algorithms and the logic to make the network card completely self-discovering in the network, self-configuring for how it actually understands that topology and forwards, and self-healing when there’s a problem.
It also is responsible for understanding and selecting the best path for the traffic to take. And it’s also responsible for taking the Ethernet packets or Ethernet frames and breaking them down into flits. And we’ll talk a little bit more about where flits fit into the picture, but the main thing is that is the secret sauce that lies underneath this that is really going to help us with handling the latency problems that I mentioned earlier.
So how the whole thing works is at let’s take this example up in the top right. I mentioned earlier that we are out of the box, a six-dimensional torus topology, six dimensional toruses are very challenging to illustrate in PowerPoint. Trust me, I’ve tried. And it’s a really good way to make you want to have a drink in the evening, but this is a simplistic version of it.
This is a four by four, two-dimensional torus. If you have any curiosity about this, please feel free to reach out to us. And we can give a little bit more education on how this really looks in our product, but this is for illustrative purposes. So let’s imagine that in this network in the top the source S just comes online, he actually will, he or she, will actually announce his information to his directly connected neighbors. All of those neighbors will actually respond back with every single bit of topology information that they have, and they will take his the new nodes information in and recalculate topology. So at that point, every single node in the network has 100% topology awareness.
From that topology awareness, every one of those will individually calculate their own set of routes to every other destination. So imagine if we had a 101 node network to make the math super simple. Every one of those nodes will calculate eight routes for every other destination. So every single node will have 800 active routes.
And so let’s imagine again, that this source S is talking to this destination and it has chosen the green path as the best. But something happens along that path, whether it be a node going out or a link going out or a cable being unplugged well, that node or CME that path will no longer be a valid path.
So as soon as signal gets back to source S that node automatically drops down to the next available active path. We do not have to wait for reconvergence or recalculation in order to figure out how to get around a problem. This is fully automated, and it happens in hardware speeds. We’re talking in nanoseconds range, right?
So about, I think about 20 to 25 nanoseconds before we recognize a path is out and we reroute that traffic. So that allows us to very quickly heal the path around any kind of a failure, which gives us a very significant advantage when it comes to the resilience of the network. Now, as I mentioned before, we are taking a, so the host sends the data to the Ethernet chip, the Ethernet chip sends an Ethernet packet or anyth that frame to our FPGA. Our FPGA takes that and chops that into what I call a subframe assembly and we’re forwarding on those things. So we’re forwarding something that’s smaller than a full Ethernet frame, which gives us some very interesting inherent performance advantage.
If you’re familiar with how traditional switch networks work, most switch networks are actually built on forwarding an entire frame of whatever that is. And especially when you have mixed traffic types, you’ll often see very different and extremely large differences in the size of the frames that get sent.
And as an example, in a regular switch network, it’s not uncommon to actually see small messages that are very latency sensitive get jammed up behind larger frames. Even if a network operator had a really good QoS policy in place, you can still end up with a 64 byte message getting stuck behind a 9K data frame on Ethernet that or a 4k frame in InfiniBand.
So there’s a lot of different possibilities for this happening. The one thing about doing QoS policies in most datacenter networks is that as soon as you set up a QS policy, based on the current understanding of the application mix, the application mix can very often change and all of a sudden, your QoS policy is no longer valid.
So a lot of times we see that a lot of data center operators just don’t bother with it. They go and essentially just run basic first in, first out or FIFA. And so what that means is that if two large packets or two large frames get to the interface before the latency sensitive small message, it’s going to have to wait for a pretty significant amount of time.
This is where we see things like tail latency coming into effect. What we are proposing to do, and what we’re doing in our current product is we take those and chop them into smaller pieces that we call flits or flow control units. So these flow control units are variable length forwarding atomic units that are variable length up to a maximum size of 160 bytes.
So what that lets us do is we can interleave the flit, in the FPGA in the transit path and at the egress interface that allows us to be a lot more fair to the latency, sensitive small packets, and also provide a decent amount of bandwidth available for the large data frame. That large frame data flows that are being communicated as well.
So this allows us to be a lot more fair for all different types and sizes and mixes of traffic. So what we’ve been seeing from a benchmark and performance perspective is that we want to do a comparison because almost any switch will run really good and almost any network as a whole will run really good if there’s no load, the problem is no production network runs well, not almost no production network runs only one single application with no other cross talk or congestive communications. So what we wanted to do was actually do something that’s much more realistic and actually do comparisons of what is latency on a network with no load, but then what is latency on a network with basically max congestion?
What we do is we set up a test bed: we built an ethernet network that was not oversubscribed, so basically the uplinks to down leaks or one-to-one, we also did one where the uplinks to downlinks for two to one and two to one is not a bad oversubscription rate, it’s actually extremely common. I’ve seen things as high as eight to one even higher than that in some cases at 12 to one. So this is not an uncommon practice to build non oversubscribed networks. I would say it’s way less common to build completely non oversubscribed fabrics.
But if we take this and we compare them, what we used was the OSU latency benchmark, and we ran some tests, run it unloaded, then run it loaded and compare. So what we’re seeing with the Rockport network is that we go from about three microseconds to about 3.5ish microseconds. So about 15 to 20% increase. On a non-oversubscribed, Ethernet network, we go from about two microseconds to over 20. So like a 10 X increase. On a two to one oversubscribed network, we’re seeing that we go from two microseconds to over a hundred. So almost a 50 X increase. The same thing is similar when we look at InfiniBand. Now InfiniBand is better at handling congestion based forwarding and lower latency forwarding, but what we’re seeing is that yes, InfiniBand is way better than Ethernet in most cases, but it still has a pretty significant impact when congestion is present.
So what we’ve seen in our testing now, this is a Rockport network compared to a 200 gig InfiniBand network running the OSU latency benchmark, is that the InfiniBand network runs around two microseconds without load and up to 10 microseconds with load. So five X increase and again, same kind of comparison in our network where we see that we go from about three, to about 3.5 microseconds of latency. So around a 15 to 20% increase in latency. Significantly better and a lot more consistent, low latency performance than we see in any other network. That’s just a benchmark. A lot of people say, Hey, benchmarks can actually be manipulated to tell the story you want to tell. What we’ve done is we’ve had some of our customers do some actual workload testing for us and give us the results to let us know if our assumptions of how this is going to impact the applications is really true.
And so you see an example here, this was actually an HPC application, which was basically a hydronic dynamics code simulation model, that basically they ran it in a couple of different ways. One of them. They ran it with no congestion. Then the other, they basically said, Hey, let’s run eight workload nodes and let’s take eight nodes and use those to basically just blast the network with full of congestion.
And so what we saw was at the best, or at the most congested, the Rockport network finished the application almost 30% faster than the InfiniBand network did. Now, what this means is that if you map this out, let’s say that this was a genome sequencer, if you can get a genome sequenced one third faster, then that’s one third faster to bring a product to market, to either be a vaccine or to be a drug for saving lives.
So this is a legitimate possible outcome that we can actually say a network can bring a product to market faster by helping the applications that are doing the modeling much quicker. So as another customer that we have did some testing using an open source, natural language process trainer using GPU’s. And what we saw was now you can see there’s a lot of different types and mixes of configurations that we used, but essentially what it came down to was: in every single case the Rockport network completed the application faster, anywhere from 19% faster, to almost 40% faster compared to the exact same servers running in a 100 gig InfiniBand.
So we’re seeing that this is actually starting to show itself in a lot of different places where we’ve actually got a fairly significant impact to the completion of applications. And what we want to do is talk a little bit about how this actually impacts the overall operations of the company.
As I said earlier, what we wanted to do is we wanted to target the traditional hotspots in a traditional switched network. So the transceivers, the cables, the switches and things that take up those really critical rack units in the data center and remove those. So the solution that we currently have actually has it.
The only thing that requires power and cooling, the only place that there’s configuration and complexity, that’s all automated sits in the cards themselves. There’s nothing else outside of that, that requires power requires configuration and logic and requires cooling. So it’s a much more ecologically friendly footprint when you compare it to any other traditional networking.
So we did some comparisons of what this would look like for a network of 288 nodes in InfiniBand and in Ethernet. And what we see is that basically within the networking components, we see a over 60% savings in power, 80%, less space required, 90% less weight, which is applicable in certain cases where, you know, especially in some mobile unit type of things planes and ships and things like that, 90%, less weight and a 75% reduction in the number of cables.
So overall it gives us a really good story, especially as companies are more and more looking to figure out how to be more green, in their really inefficient data center space. If this is something that, a CEO or a CIO has a management objective to reduce greenhouse gases from overall operations, this is something that can directly impact that type of measurable impact.
So in summary Rockport has really rethought and reimagined the network as it’s related to this performance intensive era of HPC and AI clusters. What we’re delivering to the market is a better performing network that makes data center utilization more efficient. So the clusters that are being used for these types of things, we’re actually putting more of your investment towards actually getting response and result out.
You have less complexity and a much more simple deployment and a lot more simple management. You also have the same amount of work or more work being done with a footprint that has a significant reduction in the power cooling and space required. Therefore, giving you a much greener data.
Thank you for your time, and if you want to check out any more information, hit up our website at rockportnetworks.com and you can send an email to info@rockportnetworks.com. Thanks again. Hope you all have a great day.